- Published on
Lecture Note 6: Multimodal autonomous agents
- Authors
- Name
- Lucas Xu
- @xianminx
CS294/194-280: Advanced Large Language Model Agents
Lecture Note 6 : Multimodal autonomous agents
- Web Agents
- WebArena
- VisualWebArena
- Tree Search for Language Model Agents
- Towards Internet Scal Training for Agents (InSTA)
- Questions
Web Agents
WebArena
WebArena: A Realistic Web Environment for Building Autonomous Agents
VisualWebArena
VisualWebArena: Evaluating Multimodal Agents on Realistic Visual Web Tasks
Observation:
Action:
Visual Language Models as Agents
Accessiblity tree HTML representations: cluttered with unneccessary information, long and confusing contenxt.
VLM + SoM: simplified representation with Set-of-Marks(SoM) prompting over interactable elements.
Deterministic transition function:
Baseline Agents
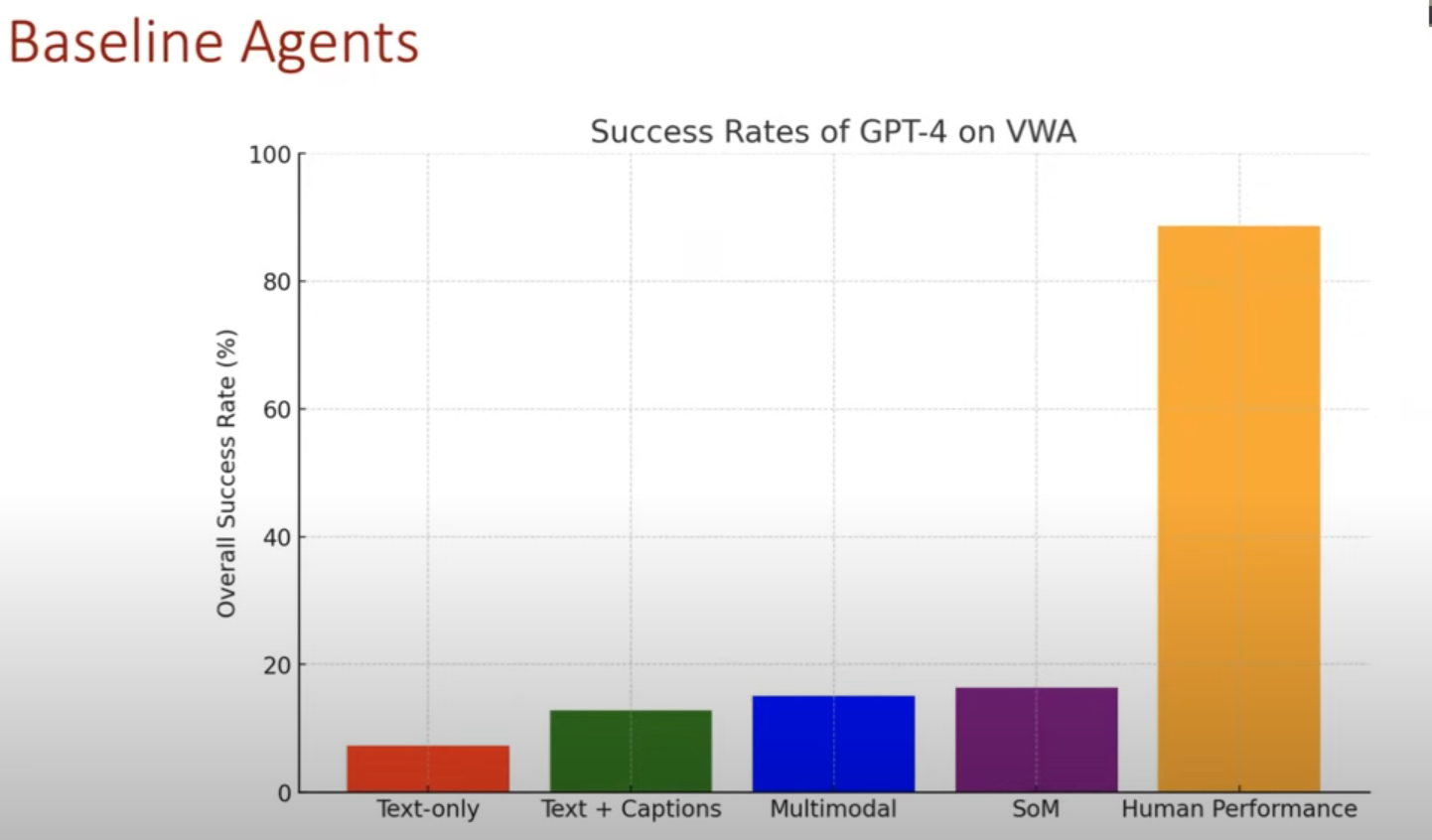
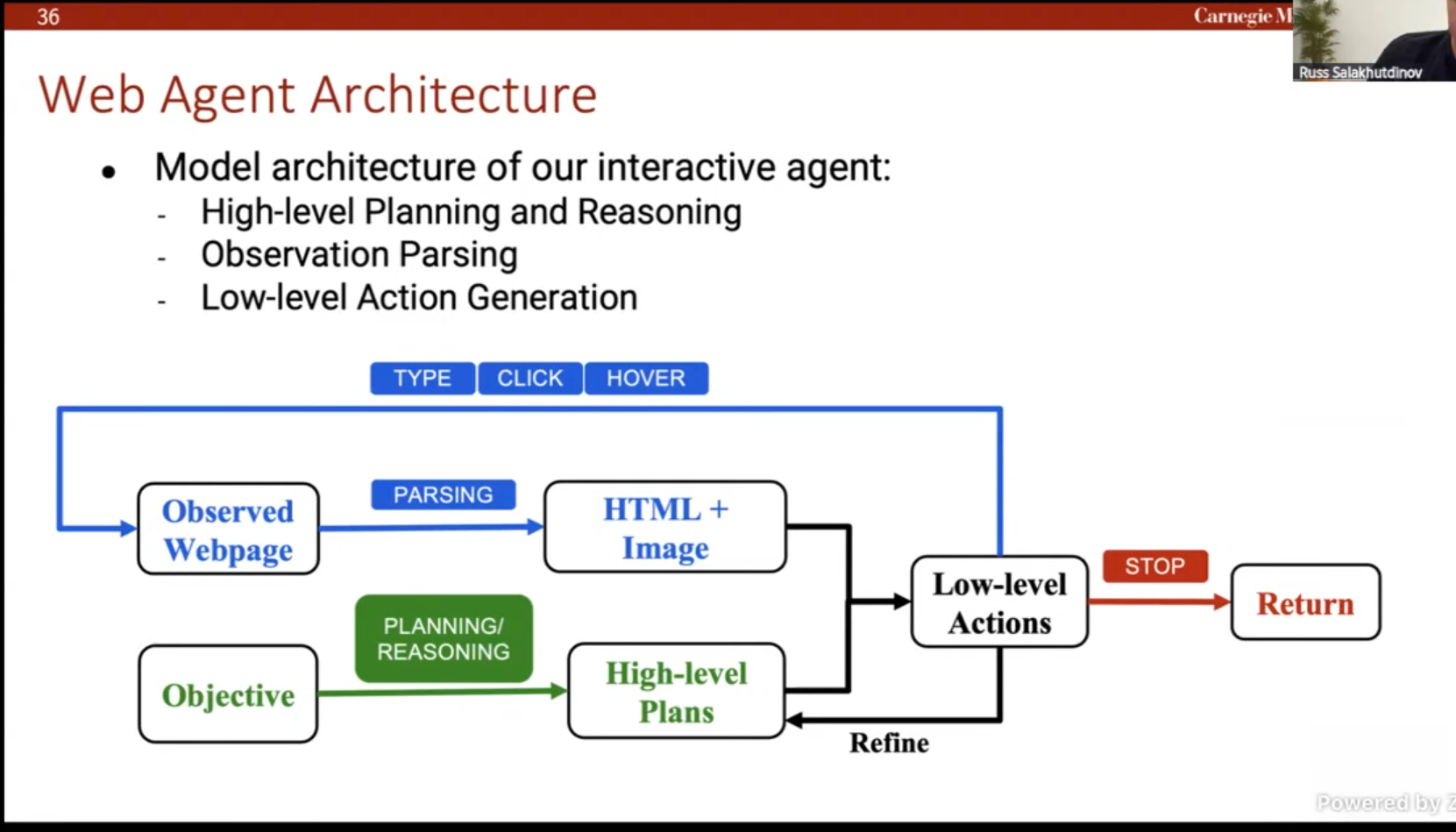
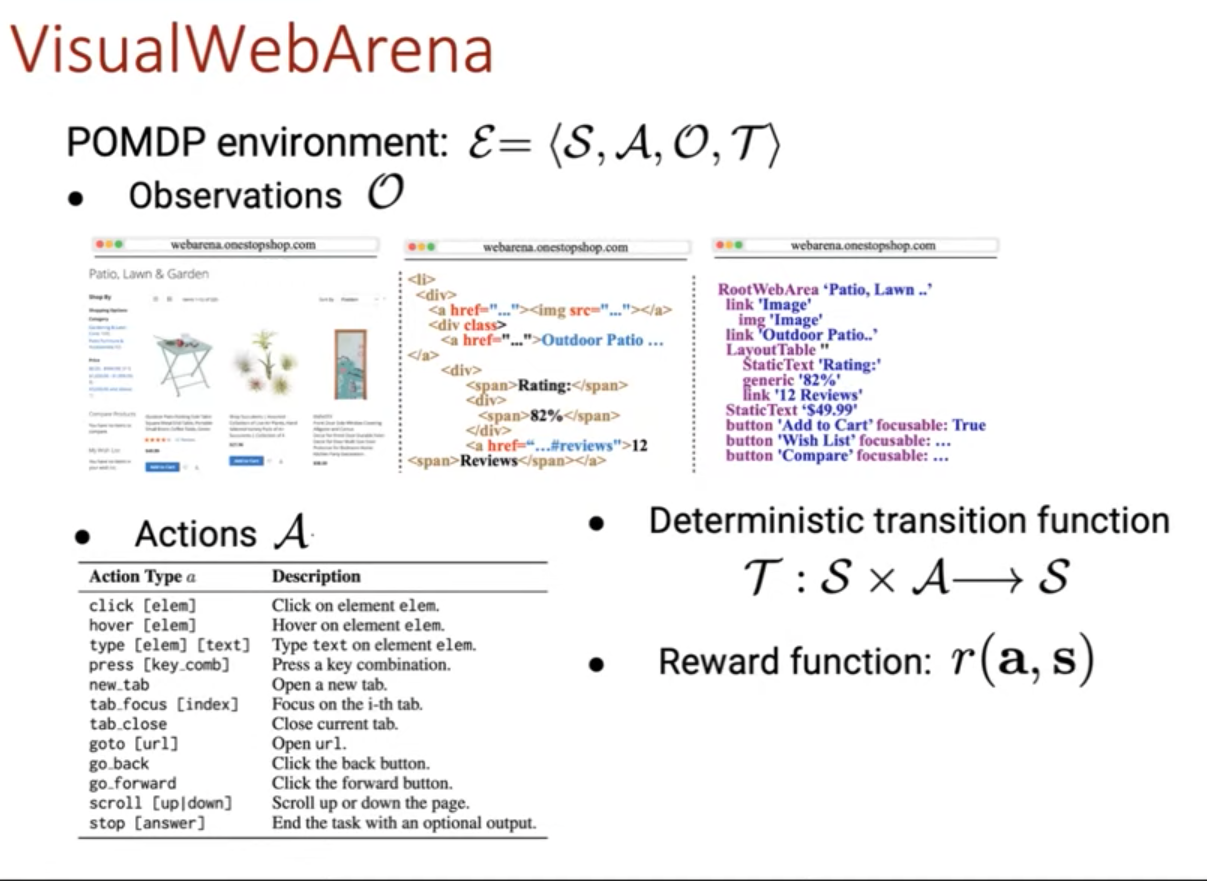
Planner:
Common Failure Modes: Long horizon reasoning and planning
Models oscillate between two webpages, or get stuck in a loop.
Correctly performing tasks but undoing them.
Failures visual
What is missing ?
We need to do a lot more to close the gaps: reasoing and planning
Measuring Productive Tasks:
Tree Search for Language Model Agents
Tree Search for Language Model Agents Jing Yu Koh Stephen McAleer Daniel Fried Ruslan Salakhutdinov
Local decisions; global consequences.
Search by repeated sampling MCTS
Key idea: apply value function to guide search.
Ablations:
Web agent and AlphaGo Zero MCTS
Limitations
- search is slow
- dealing with destructive actions
Agents suffer from data problem
Towards Internet Scal Training for Agents (InSTA)
Key Idea: use Llama to generate and verify synthetic agentic tasks
The Data Pipeline:
- Stage 1: Task Generation
- Stage 2: Task Evaluation
- Stage 3: Data Collection
Plan Sequence learn
- Planning module
Common crawl PageRank
Adversarial Attack on multi-modal agents
Questions
- What is SoM representation?

Set-of-Mark Visual Prompting for GPT-4V
The paper was published in 2023 by Microsoft. At the time, the strongest VLM was GPT-4V. The paper uses SAM employ off-the-shelf interactive segmentation models, such as SEEM/SAM, to partition an image into regions at different levels of granularity, and overlay these regions with a set of marks e.g., alphanumerics, masks, boxes. Using the marked image as input, GPT-4V can answer the questions that require visual grounding.
The method works like adding Accessiblity tags to the image.
I think this SoM works well for VLM maybe because it was training with segmentation of images.
I doubt there is no need to explicitly add SoM to the image for today's VLM.
The work was pretty simple, but very useful.
Why not use site map to assistant the navigation of the website?
what is inference time for the agent?
🔥 Turn entire websites into LLM-ready markdown or structured data. Scrape, crawl and extract with a single API. https://github.com/mendableai/firecrawl
The Lessons of Developing Process Reward Models in Mathematical Reasoning